There is a tremendous opportunity to use data safely and responsibility to the benefit of people and society. We see an increasing need for the integration and deployment of suites of tools that are interoperable and complimentary to drive the adoption of safe, useful, and timely data and analytics at scale. From our 15 years of experience working in this space, we provide an overview of the landscape of tools considered for privacy-enhancing data sharing and analytics with a view towards the needs and perspectives of different stakeholders involved in health services improvement and health research for the full lifecycle of data.
We shared our views with the U.S. Office of Science and Technology Policy to help inform the development of a national strategy on privacy-enhancing data sharing and analytics, along with associated policy initiatives (as published in the Federal Register on 06/09/2022 and available online at federalregister.gov/d/2022-12432 and on govinfo.gov). This article provides a summary of our response. For the full response, including a discussion of the data lifecycle and an analytics pipeline, see our White Paper.
Safe Data Enablement: A Spectrum of Perspectives
There are many tools for privacy-enhancing data sharing and analytics. We focus our attention on the challenges of integration and interoperability, especially for complex health data and analytical pipelines, and the impact on end users striving to improve health outcomes . Health data is often described as some of the most sensitive since it deals with the intimate details of a person’s body and mind. Once captured and collected, health data is continuously updated, transformed, harmonized and restructured to meet the multitude of needs in extracting the greatest insights from advanced statistical methods that are themselves continuously updated and improved on throughout the data lifecycle.
Data science consortia and researchers alike highlight the importance of data sharing and analytics to drive evidence-based decision making. In the highly competitive and innovative fields of health research and treatment development, solutions for data sharing and analytics must contend with data that include a large number of variables, have spatial and temporal dependence, and inconsistent or missing data (for example, due to non-response bias in surveys or complex data collection practices and linking challenges). Even static data is refactored and reharmonized to suit the various acrobatics of statistical analysis.
The 4 stakeholder types in sharing and using sensitive data that we consider represent an evolving set of perspectives, and the interplay between them is important for exploring the spectrum of tools we consider. For each stakeholder type, we include a simple “trust expression” that provides the stakeholder’s perspective on whether sensitive data will be shared and used safely and responsibly, based entirely on their role and view into the process of sharing and using sensitive data.
- End user (trust me). Responsible for making sense of data and performing analytics, starting from data clean-up and preparation, using familiar tools, and developing their own custom or tailored analysis methods. Consider the trusted professional, such as the biostatistician, epidemiologist, or a health scientist as standard examples of end users who pursue improvements in efficiencies and health outcomes.
- Risk-based IT security officer (trust, but verify). Information assets and technologies should be adequately protected, and in any modern environment there will be a role to ensure this takes place. “Risk-based” implies that the tolerance around security controls will be commensurate with the sensitivity of the information and expectations of what the end users can and should be doing.
- Privacy officer (responsibly trust). Accounting for how people represented in data feel regarding the uses of identifiable information about them. Ethics will often be a consideration, with the privacy officer concentrated on the applicable legal frameworks that attempt to codify societal norms and values into an established set of principles for the responsible sharing and use of sensitive data.(1)
- Zero-trust IT security officer (never trust, always verify). An IT architecture design principle in which a breach is always assumed and every request for data is verified as though it’s coming from outside. Even the internal transfer of data may be considered at risk. This perspective has also been introduced as a technical solution to privacy, with provable guarantees, although it doesn’t really address responsible uses of data.
These represent an evolving set of perspectives that should, in theory at least, build on one another. However, we need to go back to the beginning and ask what end users think of all these added perspectives, and how they could limit their ability to achieve their goals of delivering insights that improve efficiencies and health outcomes. While aiming to deliver trustworthy systems that address potential privacy concerns, we need to ensure that the ultimate goal of producing safe, useful, and timely data is still possible in the eyes of those professionals that derive value from working with sensitive data.
Understand the opportunities to share and use sensitive data
Find out how various tools for safe data sharing and analytics, from data derived from real people, fit across the data lifecycle. Read this 12-page white paper.
Safe Data Enablement: A Spectrum of Tools
Before we consider a spectrum of privacy-enhancing technologies, we will first look at categories in which they may be considered. This will allow us to consider the different stakeholder perspectives that were introduced, and how they may perceive these tools that are intended to address their needs.
- Trust based. Traditionally a “risk-based” approach has been used to protect confidentiality, in the form protecting information from unauthorized access. For sensitive data, this has met the needs of the end users because it usually allows them to do any preprocessing that they require prior to doing their statistical analysis, and they can get access to the analytical software tools they need for analysis (including the most recent algorithms, and customization for more advanced modelling). From the end user’s perspective, this is better termed a “trust-based” approach, since the end user is trusted to use sensitive data safely and responsibly (verified through governance).
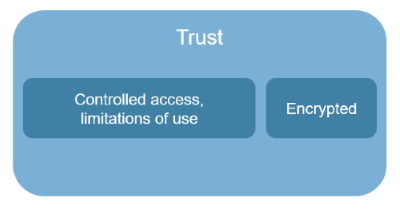
- Obfuscation based. Data minimization can reduce privacy concerns, or more advanced methods may be used to disassociate data, or remove the personal from data based on the use case, especially for purposes other than what the data was originally collected for, known as secondary purposes. Legislation to ensure non-identifiable information is used for secondary purposes is becoming increasingly common, although the HIPAA Privacy Rule has required this for large classes of health information since 2003. These are tools introduced for responsible data sharing and analytics, with a degree of trust while addressing concerns with using identifiable information.
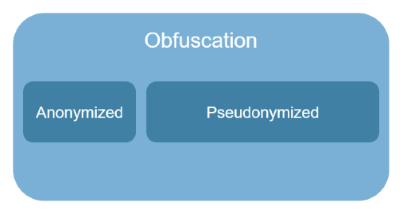
- Limited to Zero Trust. Here we begin to combine the concepts of confidentiality and obfuscation into a single category of tools. We can start with limiting trust so that the input data to statistical analyses is protected, and possibly the computations as well, since a breach is always assumed (in transfer and at the point of use). We can also extend to limiting trust so that the inputs and outputs to statistical analyses are protected. And this can be further extended to include provable security, and attempts at provable privacy (although much more complicated to define because privacy is a societal good without clear boundaries).
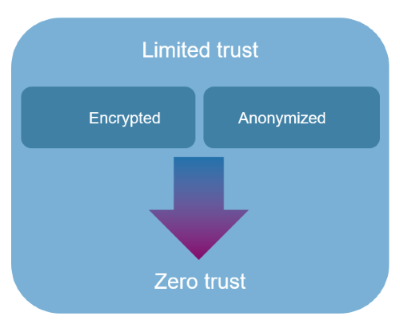
The health researcher would need to know in advance that they have clean, well formatted input data, with a limited set of predefined statistical analyses in order to use many of these tools. The reality of health data is that of complex structures, sparsity, and disparate formats that rarely line up across departments in the same organization let alone different sites. While study protocols are often defined in advance, for example to get ethics approval, they would rarely if ever define the exact statistical algorithms that will be used. In more sophisticated and established data pipelines, such as in drug development, even static data is refactored and reharmonized to suit the various acrobatics of statistical analysis.
The truth is that a great deal of analysis goes into deriving meaningful statistical results from health data: evaluating various forms of bias, understanding error distributions, inspecting outliers, testing assumptions, refining algorithms and methods, etc. While there are good examples of zero-trust tools being used, in our experience they are often academic or pilots of limited use more broadly, and ill-suited to the realities of health service improvement and research intended to meaningfully improve health outcomes.
With the above framing and description of categories, we now provide a more detailed view of the full spectrum of tools. We provide this diagram without delving deeper into each tool or subcategory, in the hopes that it will at least motivate discussion and exploration. Contact Privacy Analytics to learn more.
Conclusions
Our goal is very much to drive a conversation so that more practical and applied methods get the attention they deserve. Our hope is that the adoption of tools the enable privacy-enhancing data sharing and analytics can be increased through an increased focus on real end users that need safe, useful, and timely data and analytics that can work with the complexity and scale of real health data and modern health challenges.
In our experience, it is the entire spectrum of privacy-enhancing tools that are needed for the safe enablement of data and analytics, depending on the needs of end users and the specific uses cases being deployed. The more practical approach is therefore, in our opinion, a combination of tools rather than any one tool. While this may seem obvious to some, it bears mentioning so that more effort is put towards the integration and deployment of suites of tools that are interoperable and complimentary.
Glossary
Anonymization: transformation, including synthesis, of data with inclusion of privacy model and controls
Differential privacy: noise addition to produce indistinguishable outputs up to a defined information limit
Federated analysis: combining the insights from the analysis of data assets without sharing the data itself
Homomorphic encryption: encrypted data that can be analyzed without decryption of the underlying data
Output checking: verifying disclosure risk of analysis results conducted on confidential data
Privacy model: syntactic evaluation of data threats or formal proof of information limits
Secure enclave: isolated execution environment to ensure integrity of applications and confidentiality of assets (aka trusted execution environment, or confidential computing)
Secure multiparty computation: combining the encrypted insights from the analysis of data assets without decrypting the underlying insights themselves
Simulated data: artificially generated data by a theoretical, representative model
Synthetic data: artificially generated data by a statistical or learning model trained on real data
Tokenization: secure process of substituting sensitive data elements with non-sensitive and secure data elements